Hello, I’m Eric, the COO of applemint, a digital marketing agency in Taiwan!
As a digital marketing agency in Taiwan, we are often asked the question, “How much budget should we set?” by our clients.
I honestly think the budget should be “as much as they can afford”. Our clients have their limits in terms of budget but they still wish to know the appropriate amount of budget. Unfortunately, there are not many companies that can afford an adequate budget to properly check the effectiveness of their ads.
This is because you often need a very large sample size to see the effectiveness of your ads. In my experience, this requires a constant monthly budget of about 9,000 to 18,000 USD.
In this blog, I will explain the concept of sampling, which is one of the most important indicator when testing your ads. I will talk about why it is important to know your sample size and how to calculate it.
I hope that this blog will help you understand how to use the concept of sampling to determine how much exposure you need before you can determine the effectiveness of your campaign.
Contents
Why is it important to understand sample size?
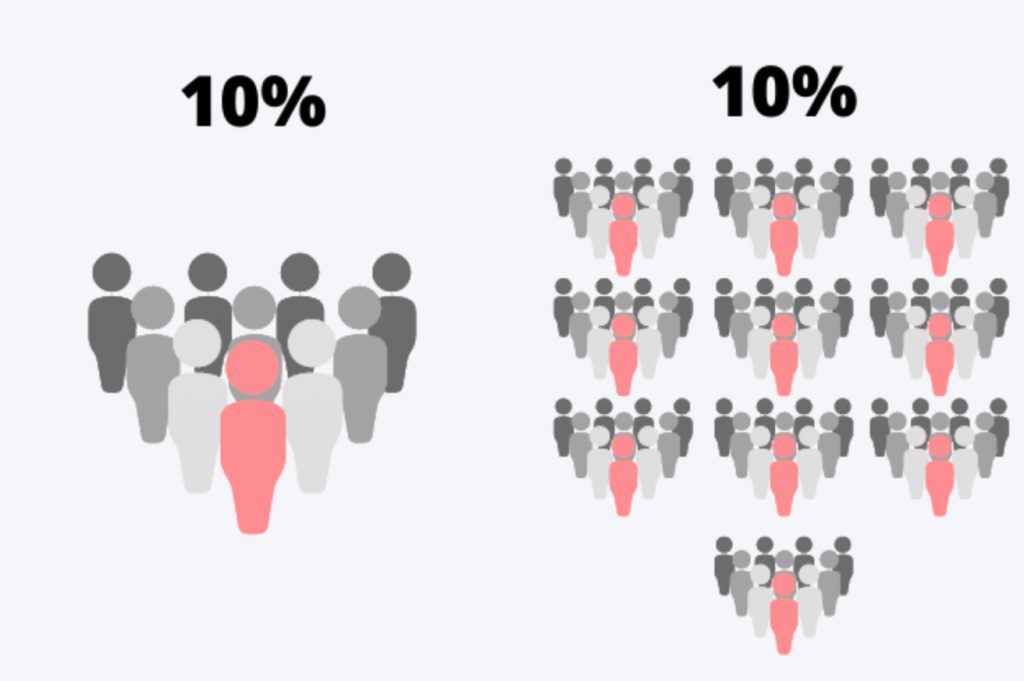
First, let’s imagine a “10% conversion rate” as a concept.
The two scenarios below both have a conversion rate of 10%, but they have completely different meanings. Let’s say we have two restaurants selling beef noodles.
- In restaurants A, 10 people came and only one bought.
- restaurants B has 100 visitors and 10 people bought.
The purchase rate is 10% for both, but which beef noodle do you think is better?
You would probably think restaurant B is better. This is because it has more visitors and so is more reliable, even though it has the same conversion rate of 10%. In this example, the number of visitors is the “sample”. In other words, the larger the sample size, the more reliable it is in representing the actual group.
When delivering online advertisements, some customers get worried when the click rate or conversion rate improves or worsens by only 0.5%. However, if the sample size is small, it means that statistically speaking, the improvement or decline is very often within the margin of error.
How do you calculate the sample size?
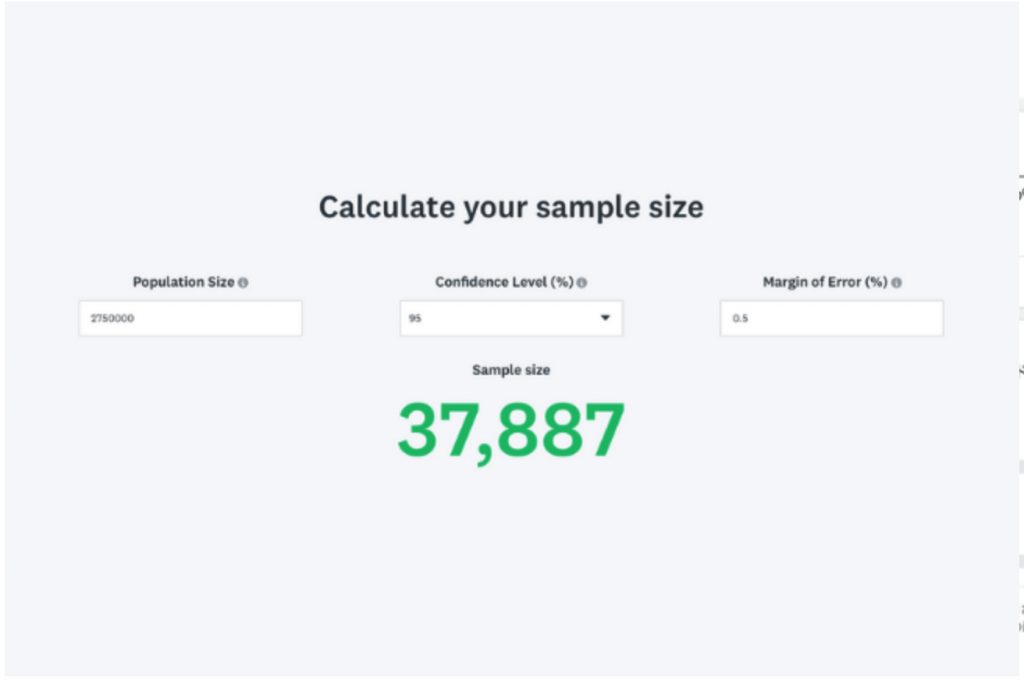
So, how large a sample size should you have? In statistics, there are formulas for determining sample size, but here we will use a tool available on the Internet to do the calculation.
The tool we are using is Sample Size Calculator provided by SurveyMonkey. When using SurveyMonkey, there are three statistical terms that you need to understand first:
1. Population
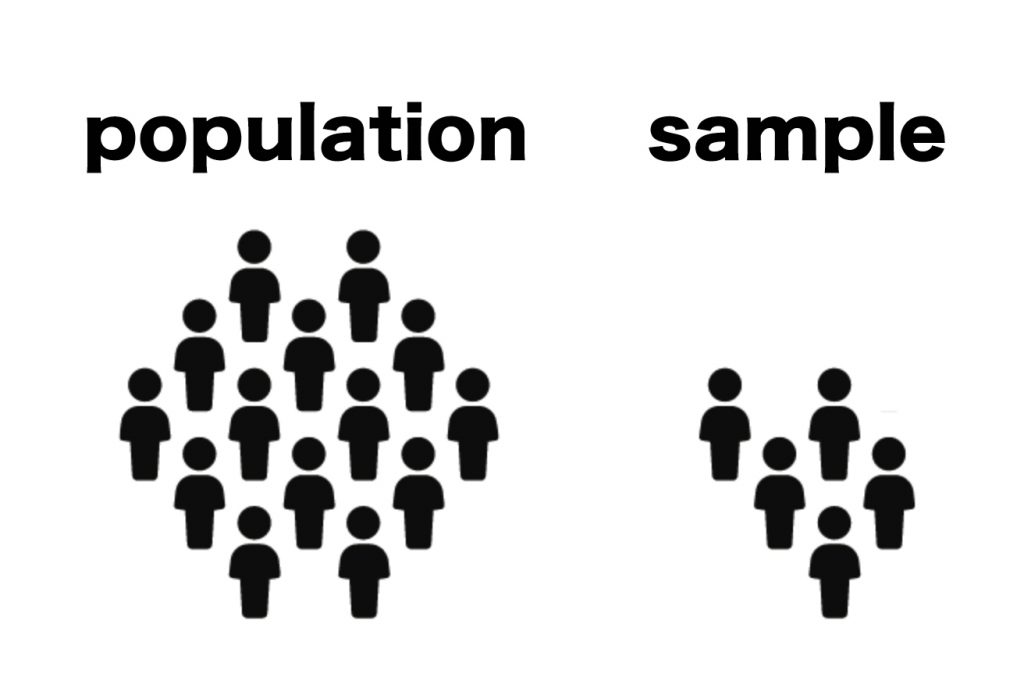
The population is the reference group from which the sample is drawn. Basically, it is the total number of groups/subjects to be tested. The sample is the group from which the test data were taken. The purpose of statistics is to estimate the characteristics of a population through the results of a sample.
Let me give you an example. In the case of a presidential election poll, the population is all citizens who are eligible to vote. Through the sampling of the poll, we can estimate the characteristics and tendencies of the population group, the voting public.
In the case of advertising, the objective is to estimate the percentage of the target population that will actually respond (click or purchase) when “exposed to all the target population” through a single advertisement.
For example, in the case of a product targeting university students and graduate students in Taiwan, the number of registered students can be estimated to be approximately 1.21 million using statistics provided by the public information platform of the Office of Universities and Colleges.
2. Confidence Level
The confidence level is the likelihood that the population (a constant representing the characteristics of the population) will be included when multiple rounds of sampling are conducted. Generally speaking, a confidence level of 95% is appropriate.
In general, 95% is used as the confidence level. Of course, if you want a lower confidence level, you will need a smaller sample size. In short, if 95% of the data in your sample is at a certain level, then you can trust the data.
In the case of digital advertising, the data on which the confidence level is based is the click rate or conversion rate. If 95% of the data is settled at a certain click rate or conversion rate, then you can conclude that the data is reliable.
The range of this data plus an acceptable margin of error is called the confidence interval. The confidence interval is the interval between the sample size plus the error. Since this acceptable error varies depending on the product or service, the data in the confidence interval will vary.
Taking advertising as an example, if the click rate for a single campaign is 2% and the margin of error is 0.5%, the confidence interval for the sample would be 1.5% to 2.5%.
Margin of Error
As the name suggests, this is the maximum margin of error that can be tolerated.
Let’s take the click-through rate of an advertisement as an example. If the click-through rate of an ad is 2%, and the data is obtained at a 95% confidence level with a margin of error of 0.5%, then we can conclude that the click-through rate falls within the range of 1.5% to 2.5%.
According to Nielsen’s TV ratings survey, the margin of error for Taiwan’s ratings is about 1.75%. In other words, the difference between a 3% viewership rating and a 1.3% rating should be considered an error.
With an understanding of these terms, let’s take a look at how they are actually used in the world of advertising.
Applying Statistics to Digital Marketing
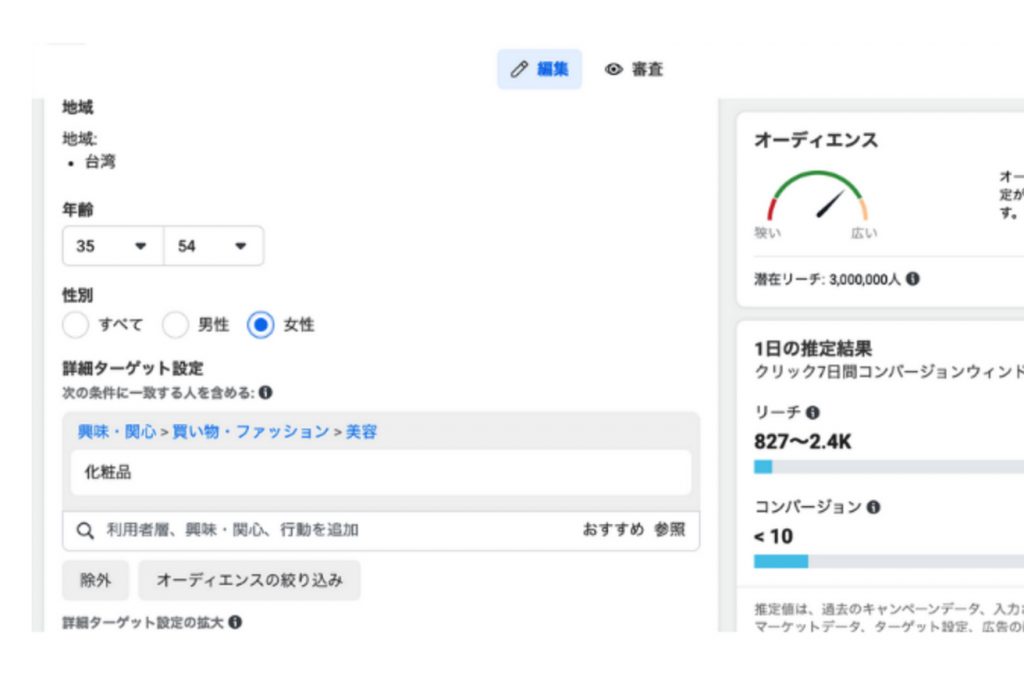
Now let’s apply the statistics we have learned so far to ditial marketing.
1) Calculating the Population
In this article, we’ll use cosmetics as an example. Facebook ads have a useful function that allows you to calculate the number of potential targets by specifying conditions using the Audience Insights Report.
In this case, we will use “Taiwanese women aged 35-54 who are interested in cosmetics” as the condition. As a result, we found out that there are about 2.5 to 3 million potential targets (population). Based on this number, we set the population as the intermediate value of 2.75 million. We input this number into the sample size calculator that we introduced earlier. The confidence level is set to 95%, which is the industry standard.
2) Sample calculation
Assuming a 1% margin of error, we now know that we need a sample of 9,571 clicks. In short, we need 9,571 clicks on our website to know if our conversion rate is reliable.
If 9,571 visits yielded a conversion rate of 1%, we could estimate that if all 2.75 million beauty hobbyists in Taiwan visited the site, we could expect an overall conversion rate of 0-2%.
3) Calculating the advertising budget
If we assume that the cost-per-click is 30-60 NTD, we would need 1-2 million NTD to get 9,571 visitors.
By the way, if you reduce the margin of error, you will need a larger sample size. For example, if you set the conversion rate error to 0.5%, you will need 37,887 visitors to your site.
Some of the companies we consult with often say, “First, we want to get a feel for the Taiwanese market with a few hundred dollars as a test. However, if your goal is to achieve a “purchase rate” or “conversion rate,” the reality is that a few hundred dollars is not even enough for a test…
Conclusion: Clarify the purpose of the test
In the world of digital advertising, so-called testing is very different from the tightly controlled environments in the natural and social sciences, where it is difficult to control many variables. As a result, it is also difficult to predict errors.
From the above calculations, we have seen how costly it is to run an ad and try to estimate the conversion rate of the entire population. So is there no value in small budget advertising?
Of course there is. In fact, “sample” and “error” can be interpreted in two different ways.
When the click rate of the advertising material is used as the test subject
Clients often express, “I want to know if this product will sell or not.” However, it is too costly to test whether a product sells or not.
Let’s say you want to test whether or not the product is interesting to potential users. Then, the test would be the ad creativity.
If you were to conduct market research on the click rate of ad creativity and ad copy, the sample you need would change from “number of site visits” to “ad reach/exposure,” and the cost would be greatly reduced.
Using the above population of cosmetic products, only 37,887 exposures would be needed to design a test with a 0.5% margin of error.
Loosen the conversion target to increase the margin of error
Second, you can significantly reduce the cost of testing by relaxing the definition of conversion and redefining it to a more general level of conversion (e.g., adding the product in the shopping cart) to increase the margin of error.
For example, in the beauty industry, widening the margin of error to 2% would reduce the testing cost to between 70,000 and 140,000 NTD (2500-5043USD).
Statistics is a “tool” in the overall marketing process and should not be an end in itself. Therefore, it is not recommended to pursue statistical significance as a goal of your experimental design.
The purpose of this blog is to help you understand the cost of error when conducting tests, and to further consider “what to test” and “how long to estimate” based on that cost awareness.
If you grasp the concept of error, you can see that it is not reasonable to treat daily reports of advertising effectiveness like a stock index and worry about it every day; unless you spend 1 million NTD a day to accumulate a lot of exposure and traffic, you will not be able to estimate the effectiveness of your ads based on the number of site visits per day alone.
That’s it, from Eric on digital marketing with statistics!
The marketing industry is in the business of selling know-how. The marketing industry is in the business of selling know-how, so in many cases people are reluctant to share their ideas and calculation models with the public.
This time, applemint has released a series of calculation models that we actually use in the field. The reason behind this is that we wanted to make these models public so that the everyone could try them out.
If you have any questions about the content, or if you think this calculation is wrong, please feel free to contact me here!
Click to contact applemint.